REAL WORLD DATA
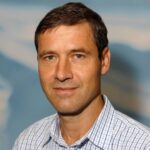
Mirek Dlouhy
CEO and Founder
October 1, 2023
(Real world) raw data is anything but consistent; It is imperfect, duplicated, outdated, and has a high degree of variability, just like everything in the real-world. However, if properly harnessed, it can power world-class analytics and insights, and help your business grow.
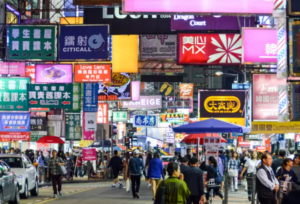
“Garbage in Garbage out” paradigm
You have often heard people use the phrase “Garbage in, garbage out” to explain the cause of poor analytics results. Is this view correct and helpful? Do they actually mean that the real world data has a high degree of variability, shifting meanings and their analytics fails to adapt to real world data?
Is it wrong to call someone “Tom”, when his legal name is “Thomas”? Would you expect that your users do not use the term “Harvard University” but its legal name “The President and Fellows of Harvard College”? Is it a duplication, if someone creates a 2nd account record with the same name and address, but in another system, e.g. CRM system? Have you made a typo today, switched letters or missed a letter? Do you have duplicate entries of the same contact on your phone? Does it hamper your phone use or even calling that contact?
Would you tell your colleague who entered a colloquial account name, like “Harvard University”, “UCLA”, that he/ she entered garbage? I have a fundamental belief that every user has the best intentions, entering accurate and meaningful data into a system. Our analytical solutions should be able to use such data, and be agnostic to natural variability.
Image 1: example of user entries for the city of South San Francisco across multiple systems
Working with real world data – humans vs computers
The examples above are just a few, reflecting how the real world (data) is; It is imperfect, duplicated, outdated, and has a high degree of variability. Despite all that, humans not only survive in such a world, but continue to strive. For humans, the variability and imperfection are usually easy to deal with. For computers and information systems, less so. At least until recently, where we have finally experienced the rise of solutions with semantic capabilities.
Real world data processing progress
It started with Google search, allowing us to find relevant information in millions of uncurated data using NLP (Natural Language Processing.) It further dramatically improved with the recent introduction of Vectorization in LLM (Large Language Models) and Vector based search engines.
Semantic functionality in enterprises
As individuals and consumers, we have benefited from advances in real-world data processing at a much faster rate than companies and enterprise system users. Even though Google search has been around for 25 years, an average IT system, such as an ERP (Enterprise Resource Planning), still often relies on exact string matches. Analytics solutions still expect that all people with a legal name “Thomas” are called that way, else it considers them to be a different person, fragmenting the analytics, data science and AI.
Using real world data – Advanced Data Mastering
Advanced Data Mastering approaches the real world data, by accepting it, producing world class analytics and insights regardless. Advanced Data Mastering focuses on processing only the required and recent data to guarantee that the data is meaningful and can be interpreted correctly (semantics). Sales to Nokia (a mobile phone company) in the early 2000s, has a completely different meaning than sales to Nokia in 2023 (a mobile network company), despite the identical linguistic name.
Advanced Data Mastering employs semantic cross-referencing and cutting-edge semantic tools to create comprehensive and precise data, which is then used to generate highly accurate analytics and data science or AI results.
Image 2: example of account names used for Pfizer accounts
“Garbage in Garbage out” postscript
The phrase “Garbage in, garbage out” is not entirely incorrect. If you fail to load all relevant data, or if the data is lost or damaged between the entry system and analytics, it will indeed result in garbage analytics.
In my opinion, it is wrong and disrespectful to call real-world data, the data entered by our colleagues into systems, “garbage.” The sooner we accept and start working with real-world data, the sooner we will be able to deliver adaptive, world-class insights, analytics, data science, and AI.
Real-world data is often messy and incomplete, but it is also the most valuable data we have. It is the data that tells us what is really happening in the world, not just what we think is happening. If we want to create truly accurate and useful insights, we need to start working with real-world data.